Artificial Intelligence and Ecommerce Product Recommendations: A Complete Guide
Artificial Intelligence and Ecommerce Product Recommendations: A Complete Guide
Short on time? We'll email you the guide to read later.
Introduction
- Artificial Intelligence (AI) refers to the simulation of human intelligence in machines, and it is being used in various applications across eCommerce.
- Personalized product recommendations can significantly increase the likelihood of a customer making a purchase.
- Popular eCommerce platforms like Amazon, Netflix, Spotify, and Etsy utilize AI-powered product recommendations to provide personalized experiences for their users.
Implementing Artificial Intelligence through product recommendations is essential for eCommerce businesses to remain competitive in today’s day and age. Understanding the benefits of Artificial Intelligence in eCommerce and how to get started is the foundational step in driving bottom-line results for your business. This guide will break down how you can utilize this technology to increase revenue, improve customer engagement, and meet evolving customer needs.
This will help you:
- Understand the concept of AI and its application in eCommerce product recommendations.
- Gain knowledge and insight on the benefits of AI-powered recommendations, the potential of AI in eCommerce, how it can improve sales and customer satisfaction, and the challenges and limitations associated with AI-powered recommendations.
Now, let’s get started!
Table of Contents
01
Introduction: What is Artificial Intelligence
02
Benefits of AI in Ecommerce Product Recommendations
03
How AI is Used in Ecommerce Product Recommendations
04
Challenges and Limitations of AI in Ecommerce Product Recommendations
05
Future of AI in Ecommerce Product Recommendations
06
Case Studies and Examples of Successful AI-Powered Ecommerce Product Recommendations
07
Personalized Recommendations in Ecommerce
How Does AI Work?
Artificial Intelligence, or AI, refers to the simulation of human intelligence in machines that are programmed to think and act like humans. This includes the ability to perceive, reason, learn, make decisions, and communicate. AI systems are designed to perform tasks that would normally require human intelligence, such as recognizing speech, identifying patterns, playing games, and driving cars.
AI has become more and more prevalent in our daily lives, powering everything from virtual assistants (like Siri and Alexa!) to self-driving cars and personalized product recommendations on eCommerce websites. As AI technology continues to advance, the benefits of AI in eCommerce are vast — its potential applications are only limited by our imagination.
AI in Ecommerce Product Recommendations
In the context of eCommerce, AI-powered product recommendations are becoming more and more common. By tailoring the shopping experience to the individual customer, eCommerce businesses can increase the likelihood of converting a customer to a buyer and offering truly personalized products.
By analyzing data such as a customer’s browsing and purchase history, demographics, and other behavioral data, AI-powered systems can suggest products that are likely to be of interest to the customer. These product recommendations can be displayed directly on the website or shared via email, push notifications, or other channels.
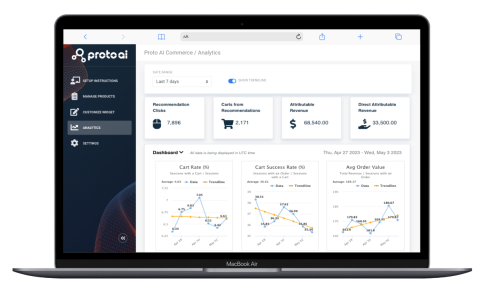
To implement AI-powered product recommendations, eCommerce businesses typically need to collect and store a large amount of data on their customers’ behavior. The data is then processed using AI algorithms to generate personalized recommendations for each customer.
AI-powered product recommendations can have a significant impact on businesses. According to Barilliance, personalized product recommendations can lead to a 70% increase in the likelihood of a customer making a purchase.
However, there are some challenges associated with AI-powered product recommendations:
- One challenge is the potential for bias in the data or algorithms, which can lead to inaccurate or unfair recommendations.
- Another challenge is the need to balance the desire for personalization with concerns around privacy and data security.
By leveraging the power of AI, businesses can provide a more personalized and engaging shopping experience for their customers, leading to increased sales and customer satisfaction. Learn more about what an AI product recommendation engine is and what to look for in this article.
Benefits of AI Powered eCommerce Product Recommendation Engines
In today’s highly competitive eCommerce landscape, providing a personalized and engaging shopping experience is crucial for businesses looking to make a name for themselves and stand out from the crowd. In fact, 52% of customers expect a personalized shopping experience that caters to their unique preferences and interests, this number is up from 49% in 2019. Especially in the case of eCommerce—where the vast array of product options can be overwhelming. This is where AI-powered product recommendations come in. By leveraging the power of AI—eCommerce businesses can provide personalized recommendations to their customers, increasing the likelihood of a sale, driving up average order value, and fostering customer loyalty.
AI-powered product recommendations are important in eCommerce for a few key reasons:
- Increased sales and revenue: One of the most obvious benefits of AI-powered product recommendations is their ability to increase sales. Suggesting products that are relevant and interesting to the customer, helps businesses increase the likelihood of a purchase and encourages customers to add more items to their carts, driving up AOV (average order value) of each transaction—54% of retailers claim that product recommendations act as the key driver of AOV.
- Improved customer satisfaction: AI-powered product recommendations improve customer satisfaction by providing a more personalized and engaging shopping experience and helping customers find relevant products faster. Customers are 2X more likely to return to a website that offers personalized recommendations that they find helpful and relevant.
- Enhanced brand loyalty: By providing a personalized shopping experience, businesses can reduce friction and encourage customers to become repeat buyers. This can have a significant impact on the long-term success of the business.
- Increased efficiency: AI-powered product recommendations can also increase efficiency by automating the process of suggesting products to customers. This can save time and resources for businesses, allowing them to focus on other initiatives.
Overall, AI-powered product recommendations are an essential tool for eCommerce businesses looking to stay competitive in today’s market. AI in product recommendations is changing the way sites operate, by providing personalized recommendations that cater to the unique needs and preferences of each customer, businesses can increase sales, improve the customer experience, enhance brand loyalty, and gain a competitive advantage.
How AI is Used in Ecommerce Product Recommendations
Artificial Intelligence, better known as AI, is an essential tool for eCommerce businesses looking to provide personalized product recommendations to their customers. There are several types of AI that can be used to power these recommendations, each with its own strengths and limitations.
Here are some of the most common types of AI used in eCommerce product recommendations:
Machine Learning
Perhaps the most used type of AI in eCommerce product recommendations—these algorithms can analyze a customer’s browsing and purchasing history to identify patterns and preferences, which can then be used to suggest products that the customer is likely to be interested in. Machine learning can also be used to optimize the recommendation algorithms over time, as the algorithm learns from user behavior and feedback.
Natural Language Processing (NLP)
This is another type of AI that can be used in eCommerce product recommendations. NLP algorithms can analyze customer reviews and feedback to gain insights into customer preferences and sentiment, which can then be used to improve the recommendation algorithms. NLP can also be used to analyze the language used in product descriptions and customer queries to provide more accurate and relevant recommendations to customers.
Collaborative Filtering
Based on the idea that people who have similar preferences are likely to enjoy similar products, collaborative filtering algorithms can analyze the purchasing and browsing behavior of customers to identify patterns and similarities. These patterns are then used to suggest products among similar customers.
Deep Learning
By using neural networks to analyze large amounts of data and identify patterns and relationships these algorithms can be used in eCommerce product recommendations to analyze customer behavior, product attributes, and other data points to provide more accurate and relevant recommendations.
There are several types of AI that can be used in eCommerce product recommendations, each with their own strengths and limitations. By leveraging these different types of AI, businesses can provide personalized product recommendations that cater to the unique needs and preferences of each customer.
How AI is Trained to Recommend Products
AI is the driving force behind personalized product recommendations in eCommerce. But how exactly does AI learn to make these recommendations? In most cases, the answer lies in the training data. While these solutions have powerful applications, they can only form predictions based on behaviors that match patterns in data they’ve previously ingested. Because of this, they require massive amounts of data to train themselves for every possible scenario they might encounter.
There are a few steps involved in training an AI algorithm—these are just the most used to train product recommendations.
Data Collection
The first step in training an AI algorithm is to collect data about a user’s past purchases, product searches, and other interactions with the platform. This data typically comes from a variety of sources, like website analytics, customer reviews, and the purchase history of your customers and is stored in a database and used as input for the machine learning algorithm.
Data Cleaning
Once the data has been collected, it must be organized to ensure that it is accurate and useful for training the algorithm. This may involve removing duplicates, correcting errors, and standardizing the data format.
Feature Extraction
This involves extracting features from the data that are relevant to making product recommendations. For example, customer behavior data may include information on which products they have viewed, how long they spent on each product page, and which products they have purchased. Product attribute data may include information on price, brand, category, and color.
Model Training
Once the data has been cleaned and features have been extracted, the AI system trains a machine learning model to predict which products the user is most likely to buy. The model may use various techniques, such as collaborative filtering, content-based filtering, or a combination of both.
Validation
Once the training is completed, the algorithm is validated using a set of test data that it has not seen before. This validation ensures that the algorithm provides accurate and useful recommendations and can be used in a production environment.
Training an AI algorithm for product recommendations typically requires a large and clean dataset, relevant feature extraction, machine learning techniques, and validation. By following these steps, eCommerce businesses can ensure that their AI algorithms are providing accurate and personalized recommendations that cater to the unique needs and preferences of each customer.
Proto AI’s proprietary Seraphim Predictive Science™ technology relies on real-time behavior, rather than training data, to make predictions. Unlike most traditional AI, Proto AI’s next generation AI makes it possible to understand what will happen in the future without the need to “train” or collect large datasets. Instead, Proto AI looks at individual behavior patterns and enriches it with contextual signals and data gathered from advanced data sampling and inference to quickly get smarter and deliver custom recommendations.
Platforms Utilizing Product Recommendations
As online retail continues to grow, more and more businesses are turning to AI to provide personalized product recommendations to their customers. Here are some examples of popular platforms that are using AI-powered product recommendations:
Amazon
Amazon is one of the pioneers of AI-powered product recommendations in eCommerce. Its recommendation engine uses machine learning algorithms to analyze customer behavior and suggest products that they are likely to be interested in. Amazon’s recommendations are based on a variety of factors, including the customer’s browsing and purchase history, product ratings and reviews, and the behavior of similar customers.
Netflix
Netflix uses a similar approach to recommend movies and TV shows to its users. The platform analyzes a user’s viewing history and provides personalized recommendations based on their preferences.
Spotify
Spotify uses machine learning algorithms to recommend music to its users based on their listening history and preferences. The platform also takes into account factors such as the time of day and the user’s location.
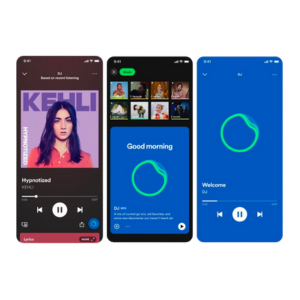
Etsy
Etsy is an online marketplace for handmade and vintage items. Its recommendation engine uses machine learning algorithms to analyze customer behavior and suggest products that are relevant to their interests. Etsy’s recommendations are based on a variety of factors, including the customer’s browsing and purchase history, product attributes, and the behavior of similar customers.
These are just a few examples of the many eCommerce platforms that are using AI-powered product recommendations to provide personalized experiences for their customers. By leveraging the power of AI, these platforms are able to provide relevant and accurate product recommendations that cater to the unique needs and preferences of each customer.
Challenges and Limitations of AI in Ecommerce Product Recommendations
Ethics
One of the key challenges and limitations of using AI in product recommendations is the ethical considerations that come along with it. Bias is a major concern—AI systems can be trained on biased data, which can lead to recommendations that are unfair or discriminatory. For example, if an AI system is trained on data that is biased against certain groups, like certain races or gender, it may recommend products that are not relevant to those groups leading to a loss of trust to the affected groups. To address the issue systems must be regularly audited to identify and correct any biases that may occur.
Privacy is another major concern when it comes to using AI—systems collect and analyze a vast amount of personal data about users, including their browsing and purchasing history, which can be used for targeted advertising or other purposes. This can be a violation of users’ privacy and can lead to concerns about data security. Ensuring that AI systems are compliant with data privacy laws and regulations, such as GDPR and CCPA is critical. Unbiased, transparent, and privacy-compliant product recommendations help build trust with their users and provide them with valuable, personalized recommendations while respecting their rights and privacy.
Technical Issues
When it comes to product recommendations, data quality can be a technical challenge. One of the most common technical issues is the cold start problem that occurs when a new user or product is added to the system, and there is no data available about their preferences. Most AI systems require large amounts of high-quality data to accurately learn user behavior and preferences. To address the issue, platforms must ensure that their data is clean, consistent, and representative of their user base.
Model accuracy is another technical challenge—AI models must be trained on relevant and up-to-date data to provide accurate and effective recommendations. However, the accuracy of these models can be impacted by various factors, such as the complexity of the model, the quality of the data, and the variability of user behavior.
AI has the potential to transform eCommerce product recommendations, it also poses a number of technical challenges that must be addressed.
Limitations
AI can analyze large amounts of data and identify patterns but understanding human preferences and behavior can be tricky.
Human preferences and behavior can be influenced by a wide range of factors like personal taste, cultural background, and social context. An AI algorithm might be able to identify that a customer has purchased a lot of products from a particular brand, but it might not be able to understand why the customer likes that brand.
Human feedback can help improve the accuracy of recommendations and can then be used to improve the algorithms that are used to make recommendations. Businesses can minimize the impact of the limitations of AI and improve the accuracy and relevance of their recommendations.
Future of AI in Ecommerce Product Recommendations
The future of AI in eCommerce product recommendations is exciting and full of potential with the constant advancements and innovations in tech. As AI evolves and matures, eCommerce businesses can begin to leverage new capabilities to provide more personalized and effective product recommendations to their users.
eCommerce platforms can leverage emerging trends in AI, to provide greater transparency and accountability in their product recommendations. By enabling users to understand the reasoning behind AI-generated recommendations, they can build greater trust and engagement with their users.
Successful AI-Powered Ecommerce Product Recommendations
There are several platforms that have leveraged AI-powered product recommendations to drive revenue and customer satisfaction. These platforms have implemented AI techniques to provide personalized and targeted product recommendations to their users, leading to increased engagement, higher conversion rates, and improved customer satisfaction.
Amazon’s recommendation engine, which is based on machine learning algorithms, analyzes user behavior and purchasing history to provide personalized product recommendations to each individual user. As a result of these personalized recommendations, Amazon has been able to increase sales and customer engagement.
Netflix’s recommendation engine, which is based on deep learning algorithms, analyzes user behavior and preferences to provide personalized movie and TV show recommendations to each individual user. This personalized approach to content recommendations has helped Netflix to retain its customers, increase engagement, and grow its user base.
Stitch Fix’s recommendation engine, which is based on machine learning algorithms, analyzes user feedback and styling preferences to provide personalized clothing recommendations to each individual user. This personalized approach has helped Stitch Fix to increase sales, improve customer satisfaction, and grow its user base.
Proto AI is different by design — proprietary Seraphim Predictive Science™ technology has reimagined the way artificial intelligence works by relying on real-time behavior unlike traditional AI to make predictions. The mission of Proto AI is to reshape the way businesses operate with AI solutions that help them make better, smarter decisions, faster to drive more revenue and create more personalized customer journeys.
— Article Continues Below —
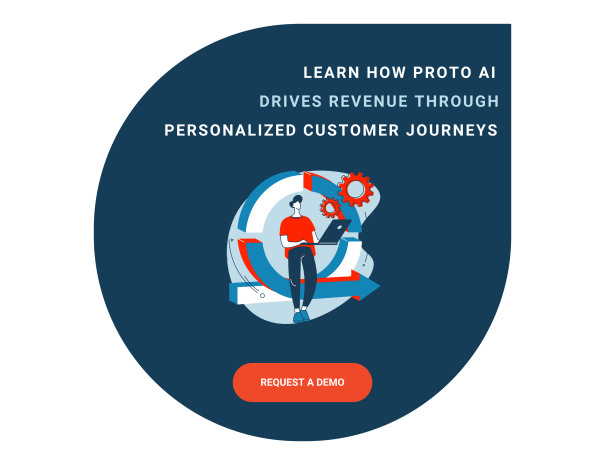
The impact of AI is significant—providing personalized and targeted product recommendations allows businesses to improve customer engagement, increase conversion rates, and drive sales. AI-powered product recommendations also help improve customer satisfaction, providing users with a more personalized and relevant shopping experience.
Personalized recommendations in Ecommerce
The benefits of AI-powered product recommendations in eCommerce include increased revenue, higher customer engagement, and improved customer satisfaction through more personalized and relevant shopping experiences. AI techniques such as machine learning and deep learning can analyze user behavior and preferences to offer targeted recommendations.
Despite the challenges and limitations associated with AI, the future of product recommendations is promising with constant advancements and innovations in capabilities. Businesses that implement AI-powered product recommendations can gain a competitive edge in the industry but must remember the ethical implications and work on addressing technical challenges that may arise.
As an eCommerce business, implementing AI-powered product recommendations is an essential step to remain competitive and meet the evolving needs of your customers in today’s digital age. Proto AI’s mission is to deliver a better experience for customers, leading them to spend more and purchase more items, and better results for retailers who experience higher conversion rates, stronger revenue growth, and less inventory spoilage. Proto AI requires very little data to function, making it easy for companies large or small to get started quickly and see value in days, not months.
Implementing AI-powered product recommendations is a key strategy for eCommerce businesses looking to improve revenue, customer engagement, and satisfaction. With the right tools and approach, businesses can leverage AI to provide a more personalized and effective shopping experience for their customers, leading to greater success and growth in the future.